UCGIS I-GUIDE 2023-2024 Community Champions Cohort
UCGIS I-GUIDE Community Champions expand the community reach of I-GUIDE. This year, the Community Champions will contribute to refining, enhancing, and applying the I-GUIDE Convergence Curriculum for Geospatial Data Science.
The Convergence Curriculum for Geospatial Data Science is a flexible and integrative educational framework designed to help students, scholars, and professionals tackle complex real-world problems. It focuses on building foundational knowledge through five key threads: ethics, analytics, geospatial concepts, computing, and visualization.
As individuals progress through the curriculum, they integrate skills and knowledge through culminating in a convergence of competencies and domain expertise. The curriculum offers various depth levels, allowing customization for different learning needs, and is continuously refined based on community feedback and evaluation, with new content releases planned for 2023.
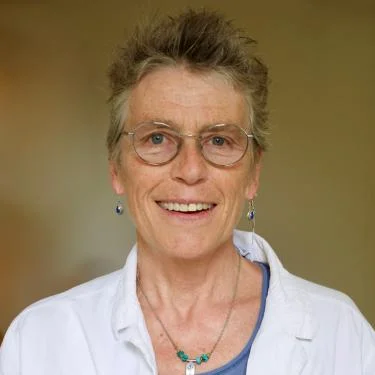
Visualizing Uncertainty in Geospatial Data
Barbara "Babs" Buttenfield
Professor Emerita, Department Geography
University of Colorado, Boulder
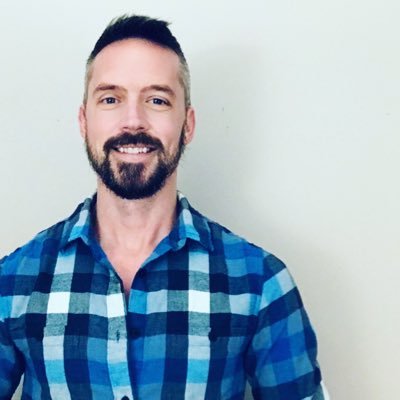
Case Studies in Convergence: Deconstructing Geospatial Connections to Frame Convergent Problems Across Disciplines
Eric Money
Associate Director of Educational Innovation, Center for Geospatial Analytics
North Carolina State University
Through collaboration with the Data Science Academy, and The Colleges of Natural Resources and Humanities and Social Sciences at North Carolina State University, this project will leverage the convergence curriculum for geospatial data science for two main goals. First, we will identify two convergent case studies, one from the natural/physical sciences, and one from the social sciences. Second, these case studies will serve as the basis for the development of instructional materials meant for a broad student audience just getting familiar with geospatial data science. The case studies will be ‘deconstructed’ into each of the individual threads, connections, and frames of the convergence curriculum to illustrate specific data, tools, and analytical strategies that can be deployed to address that case study’s convergent problem. Through this work we hope to provide a practical application of the convergence curriculum and show its utility across disciplines.