GeoAI-enhanced Community Detection on Spatial Networks Using Graph Neural Embeddings
February 19, 2025 11:00 am (Central Time)
Recorded VCO
Abstract
Spatial networks are essential for modeling geographic phenomena influenced by spatial interactions. Traditional graph-based community detection methods identify strongly connected components but often overlook node attribute information. This presentation will describe region2vec, a family of GeoAI-enhanced unsupervised community detection methods using graph neural networks (GNNs). Region2vec generates node embeddings based on attribute similarity, geographic adjacency, and spatial interactions, then applies agglomerative clustering to extract spatial communities. These methods optimize both node attribute similarity and spatial interaction intensity, making them valuable for applications like health service area delineation, ecological zoning, transportation planning, and political redistricting. The developed models will be hosted on the I-GUIDE platform for interdisciplinary use.
Speakers
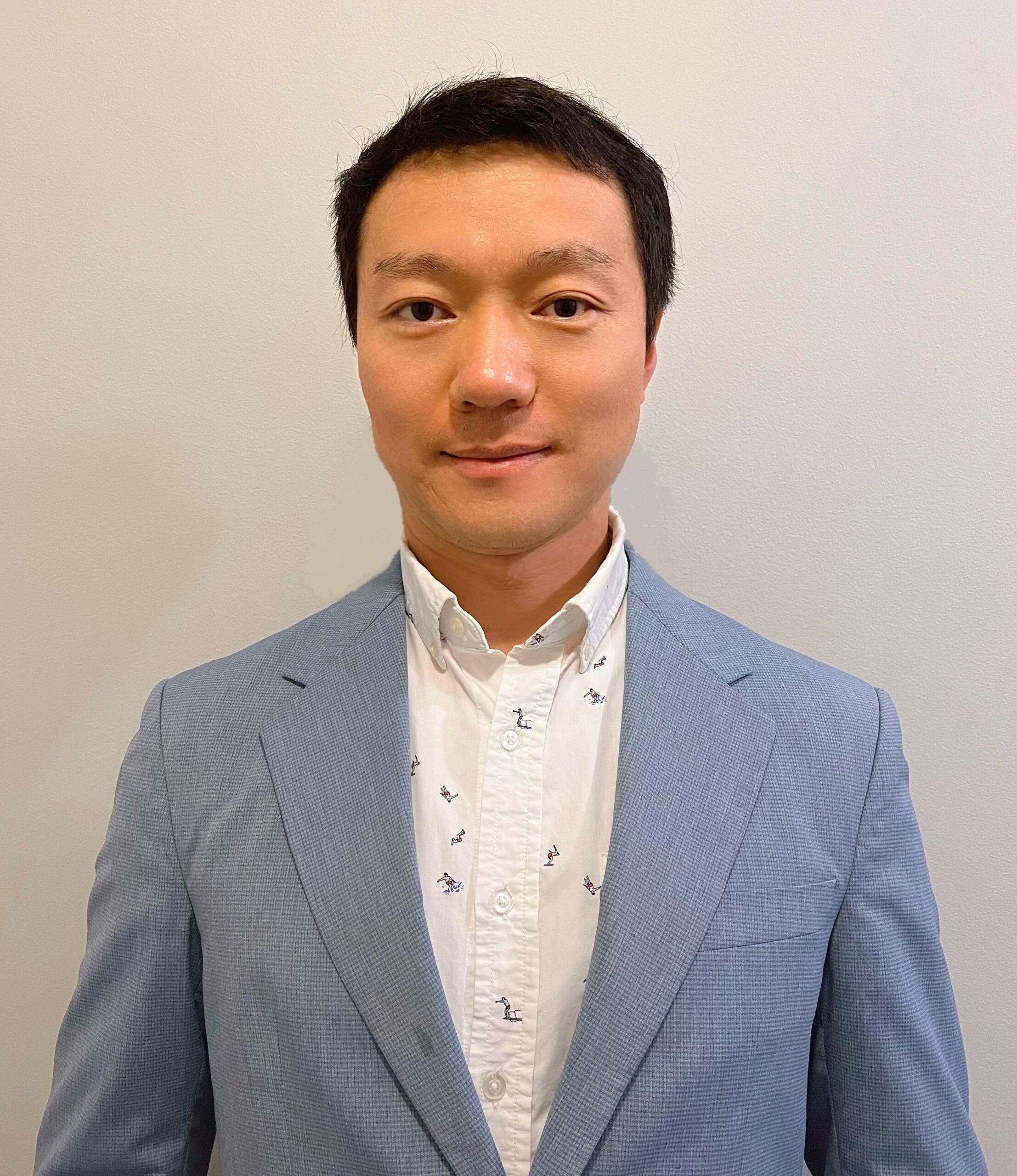
Song Gao
University of Wisconsin - Madison
Dr. Song Gao is an Associate Professor of GIScience in the Department of Geography at the University of Wisconsin - Madison. He uses spatial statistics and machine learning to increase understanding of human mobility patterns and human-place interactions. He develops and applies geospatial data science methods for better sensing of human behavior, socioeconomic characteristics, and urban dynamics.